Causaly, Machine Reading and the Future of Biomedicine
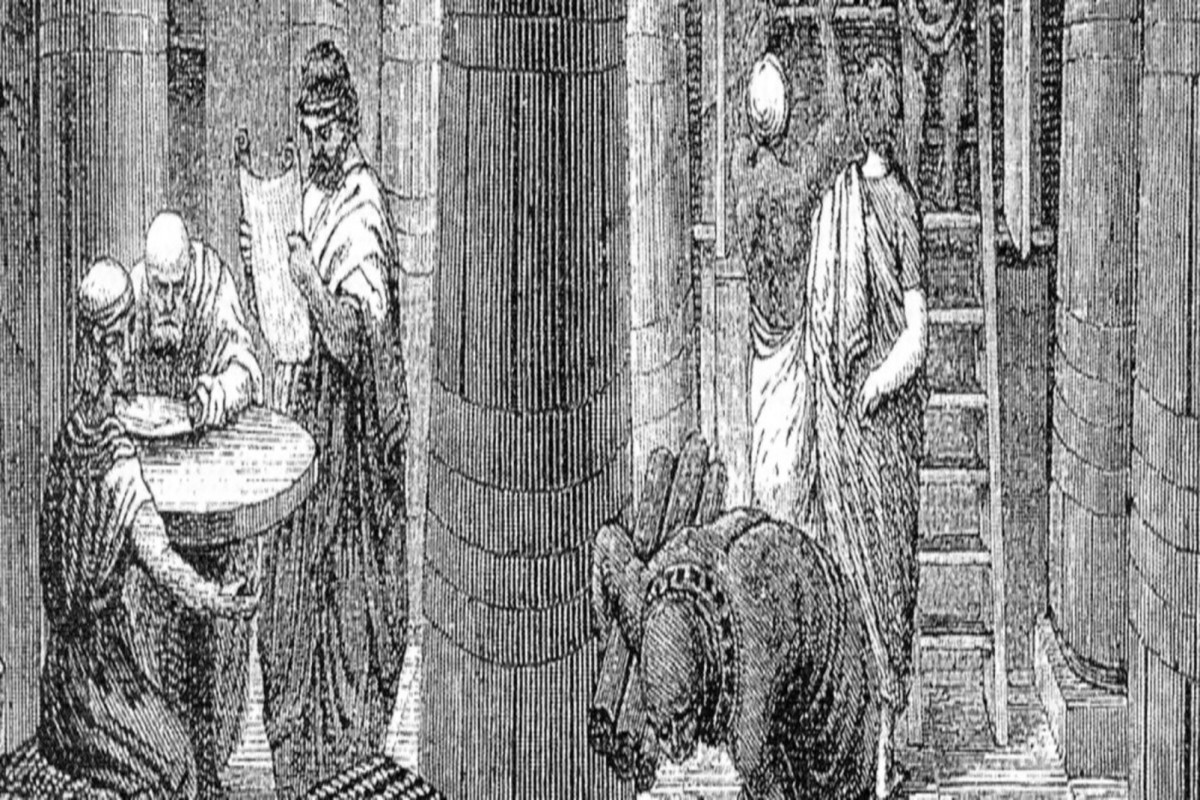
A scholar walking into the Library of Alexandria in 200 BC –the ultimate repository of human knowledge at the time– would search for a book, an author, or a topic; at best, they would also reach out to the librarian for some guidance. Essentially, they were looking for one or more ‘keywords,’ ranking the results according to a ‘popularity’ metric.
Fast forward to today; our generation’s repositories of human knowledge have become massive, and digital. Their volume is growing exponentially, and we can get immediate access remotely to every bit of them. Still, in its essence, researching scientific literature has not changed; we are still looking for keywords, ranking the results according to a popularity metric.
However, a text is not just a random set of words; it also accommodates a set of fundamental relationships between them. Linking plain keywords and documents with each other and aggregating ‘votes’ between them does not capture any underlying causality. As a matter of fact, reading comprehension remains a task reserved for humans, and we have started to realize the limitations.
Now, what if one could search for relationships between entities instead of raw keywords? What if you could discover the causes and effects behind words, also navigate between them? What kind of opportunities would such a development introduce?
We discovered Causaly when visiting Berlin last year and had a first chat with Yiannis Kiachopoulos soon after that. We were fascinated by what we saw.
A few months and a few captivating meetings later, Yiannis and his cofounder Arthur Saudabayev had moved to London and we were excited to become part of the company’s mission.
Causaly starts from Biomedicine, building a knowledge graph that captures relationships between disorders, chemicals, drugs, and genes. Leveraging published academic literature, it currently features more than 100 million causal associations.
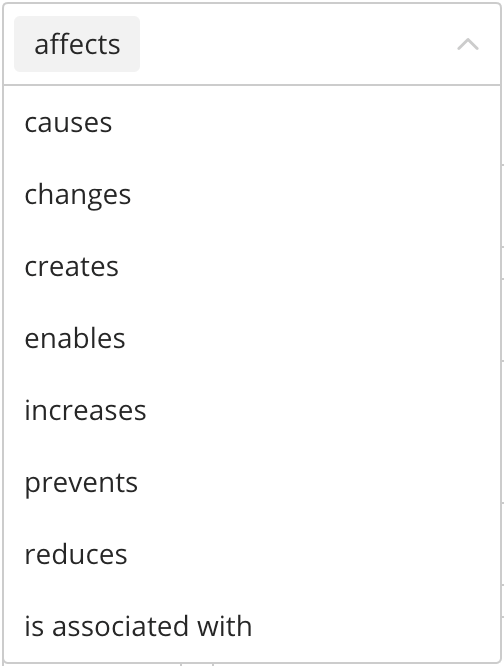
Enormous volumes of assets are getting wasted in pharma and the life sciences by not fully leveraging past learnings and data. To give an example, a new drug takes at least ten years and one billion dollars to develop, and it turns out that in many cases people are looking for an answer in the wrong places to begin with…
Swiss Novartis is Causaly’s first prime customer, followed by an array of pharma and biotech companies that are leveraging the platform to accelerate their execution. Their researchers are now empowered to investigate complicated research questions across thousands of documents and understand how diseases work or develop novel research hypotheses. The days when you needed to spend months ‘performing’ literature reviews, instead of contemplating their outcomes, should be limited.
Causaly is leveraging the latest natural language processing and machine learning developments to build its cutting-edge technology and provide access to it via a highly practical and visually appealing interface. Naturally, it collaborates with strong research labs on the topic, including UCL, King’s College London and AUEB’s NLP group. If you are excited about such problems and have a strong background in computational linguistics, causal inference, machine reasoning or plain ‘old’ yet quite demanding front-end development, reach out to the team!
In Marathon, we love working with ambitious technical founders, building infrastructure technology that opens up new ways to do core tasks with unprecedented efficiency. The work of Yiannis, Artur and their team is a prime example, and we look forward to where the next steps of Causaly will bring us!